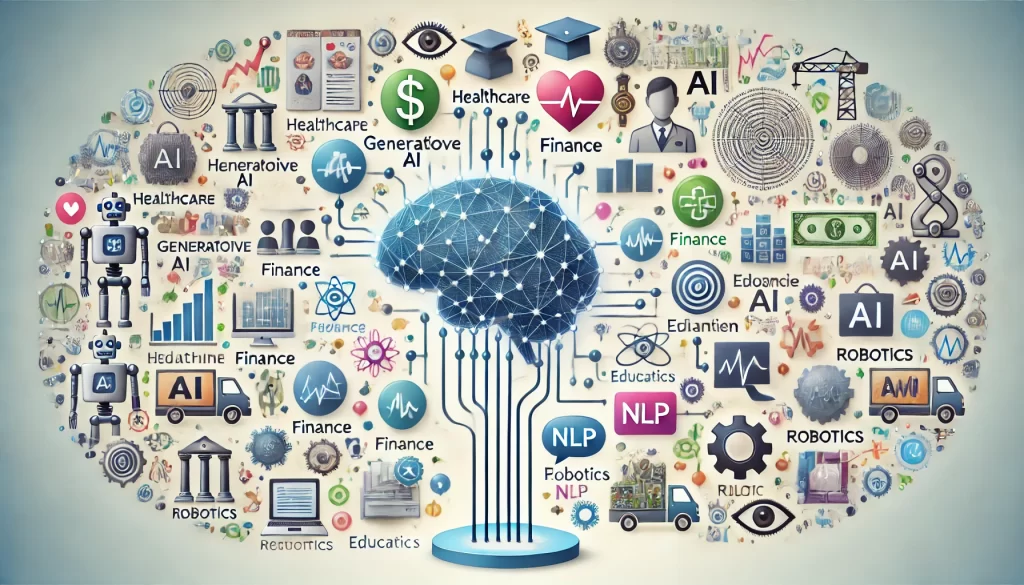
Integrating generative AI with other AI models and applications can enhance their capabilities and create more comprehensive and effective solutions. Here are several ways this integration can be achieved:
- Natural Language Processing (NLP):
- Chatbots and Virtual Assistants: Integrative generative AI can create more human-like and contextually aware responses, improving user interaction and satisfaction.
- Text Summarization and Translation: Combining generative AI with existing NLP models can improve the accuracy and fluency of summaries and translations.
2. Computer Vision:
- Image Generation and Enhancement: Generative AI can be used for creating high-quality images from text descriptions, improving image resolution, and filling in missing parts of images.
- Object Detection and Recognition: Integrating generative models can help in generating synthetic data to train and enhance object detection models.
3. Healthcare:
- Medical Imaging: Generative AI can enhance medical images, assist in creating synthetic medical data for training purposes, and improve diagnostics by integrating with existing imaging analysis models.
- Personalized Medicine: By generating patient-specific simulations and treatment plans, generative AI can assist in precision medicine efforts.
4. Finance:
- Fraud Detection: Generative models can simulate fraudulent transactions to improve the training of detection algorithms.
- Financial Forecasting: Integrating generative AI with predictive models can enhance scenario analysis and risk assessment.
5. Entertainment and Media:
- Content Creation: Generative AI can assist in creating music, art, and writing, augmenting the creative process and providing new tools for artists.
- Game Development: It can be used to create characters, dialogues, and scenarios, enhancing the gaming experience.
6. Education:
- Tutoring Systems: Combining generative AI with educational models can create personalized learning experiences, generating tailored content and feedback for students.
- Content Generation: Automating the creation of educational materials, such as quizzes and study guides, based on curriculum data.
7. Robotics:
- Behavior Simulation: Generative AI can simulate various robotic behaviors in different scenarios, improving the robustness of robotic models.
- Human-Robot Interaction: Enhancing the interaction by generating more natural and context-aware responses from robots.
8. Data Augmentation:
- Training Data Generation: Generative models can create synthetic data to augment training datasets, improving the performance of machine learning models.
- Anomaly Detection: Generating normal behavior patterns to help identify deviations and anomalies more effectively.
9. Personalization and Recommendation Systems:
- Content Personalization: Generative AI can create personalized content recommendations based on user preferences and behavior.
- Dynamic User Interfaces: Generating adaptive and personalized user interfaces that change based on user interactions and preferences.
Integrating generative AI with other AI models and applications requires careful consideration of data quality, model training, and ethical implications to ensure the effectiveness and reliability of the integrated solutions.