Source – forbes.com
This week a report by PwC found that artificial intelligence could add as much as $15.7 trillion – around same as the combined output of China and India – to the global economy by 2030.
Everyone is in agreement that it is a technology which can change the world, and from space exploration to countering terrorism and even creating art, its potential is becoming increasingly apparent.
It still faces significant hurdles though – challenges that will have to be overcome before that potential can be achieved. Many see meeting those challenges as a task of utmost priority for the tech industry right now.
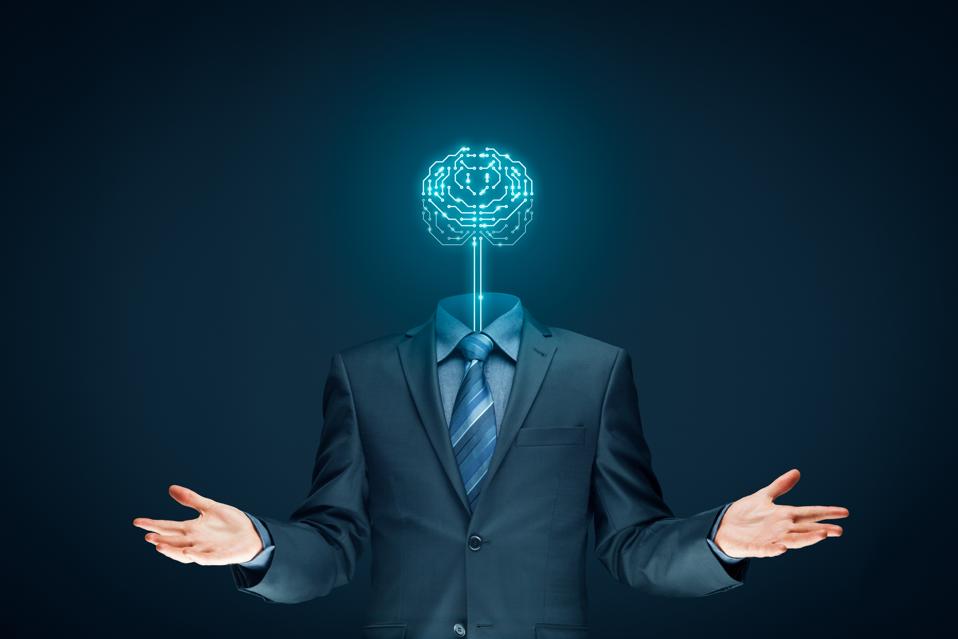
Shutterstock
Lack of compute power
Let’s start with an easy one, and one which is likely to be solved over time, although until it is, it shouldn’t be taken for granted that it will be.
AI – specifically the machine learning and deep learning techniques which show the most promise, require a huge number of calculations to be made very quickly. This means they use a lot of processing power.
Stephen Brobst, CTO at Teradata, tells me “Until about two years ago there was a brick wall – AI has been around in theory for a long time, but had been in this kind of AI winter because everyone had good ideas but they were all theory and there wasn’t enough compute power to implement them, so who cares?”
Cloud compute and massively-parallel processing systems are what have provided the answer in the short term. But as data volumes continue to grow, and deep learning drives the automated creation of increasingly complex algorithms, the bottleneck will continue to slow progress.
The answer is likely to lie in the development of the next generation of computing infrastructure, such as quantum computing, which harnesses subatomic phenomena such as entanglement to carry out operations on data far more quickly than today’s computers.
“In reality, we are at least five, more likely 10, years from that”, Brobst tells me. “We have to figure out the programming models, because the programming models for quantum are completely different from those we use now – there’s got to be a reinvention and that’s going to take time.”
Lack of people power
Until very recently, AI has been something talked about by science fiction writers and worked on in the depths of university IT research labs. In other words, without mass market use cases there has not been a great deal of money in it (unless you are making Hollywood films about robots taking over the earth.)
This means there have been comparatively few organizations willing to put money into development of these skills, and the subject was not well-represented in industry-focused education and training curricula.
With the explosion of interest in the last few years, all this has changed. Data science courses focusing on the core skills needed for AI development – mathematics, computer science and statistics – have become prevalent and are generally over-subscribed.
But there are still not enough people to enable every business or organization to unleash their vision of machine-powered progress on the world. Just as in other areas of science and technology there is a skills shortage – simply not enough people who know how to operate machines which think and learn for themselves.
Several forces are at work which should act to remedy this situation, given time. One is the emergence of what is often described as the “citizen data scientist”. There are professionals who, although not formally trained or primarily employed as data specialists, develop practical competency at working with data and analytics, usually to advance their work in their own specialized field.
Another is the move towards providing platforms and tools which enable AI-driven work “as-a-service”. Rather than having to build everything from scratch, organizations are increasingly able to take ready-made solutions and simply plug in their own data – harvesting the results which ignoring the technical operations going on “behind the scenes”.
Building Trust
Brobst predicts that by 2020 there will be a revolt by a “noisy 10 per cent” against the hold AI has taken over our lives. “The problem is that AI is a black box – people don’t feel comfortable when they don’t understand how the decision was made.
“For example algorithms used by banks are mainly linear maths and it’s pretty easy to explain the path from the input to the output – ‘I denied your mortgage application because, you don’t have a job, or whatever…’
“With multi-layer neural networks, the average human doesn’t understand, so now we’re making predictions based on things that people don’t understand and that’s going to make people uncomfortable.”
Although this revolt is more likely to take the form of social media campaigning and boycotts, than smashing machines and burning down assembly plants, it’s a hurdle which could derail attempts to drive progress.
The solution here is letting people see that this technology works, Brobst suggests. “The reality is that there’s great opportunity to make things better by having more accurate predictions, and prescriptions.
“We’ve got to get humans to understand and accept those recommendations – but that doesn’t mean to say we should never challenge machines, because we might still know something that they don’t.”
Legislation, which has so far failed miserably to keep up with the speed of technological progress, is likely to play a part in this. Growing consumer awareness of the growing number of decisions made by machines, using our own personal data, has prompted lawmakers to tackle the problem from our (the consumer’s) point-of-view. One example is the GDPR, which will come into force across the EU next year (and affect anyone dealing with the private data of EU citizens, wherever they are in the world.
This raises issues of government overstep, too, thought, says Brobst. For example a part of the regulation suggests that citizens could have the right to have an explanation for decisions which are made about them by AI.
“Under a very strict interpretation of the GDPR I can demand that, say, Netflix, explains why it recommended that movie to me.
“For Netflix this is highly confidential, proprietary, cutting-edge stuff that it has spent a lot of time and money developing. To me it doesn’t seem reasonable that if I invest gazillions of dollars building a recommendation engine, anyone can steal it from me.”
One-track minds
A final challenge which is worth considering is that the vast majority of AI implementations in use today are highly specialized.
Specialized AI, often referred to as “applied AI”, is created to carry out one specific task and learn to become better and better at it. It does this by simulating what would happen given every combination of input values, and measuring the results, until the most effective output is achieved.
Generalized AI – such as that powering robots like Star Trek’s Data, capable of turning their hand to any task just as a human can, will still be a science fiction dream for some time yet.
As Raja Hadsell, AI research scientist with Google, says, “There is no neural network in the world, and no method right now that can be trained to identify objects and images, play space invaders and listen to music.”
The problem here is that “naturally” intelligent organisms like humans are capable of taking into consideration learning and data from tasks other than the one we are currently working on. This ability to draw on resources other than those which are immediately apparent, in order to tackle a problem, is known by clichés such as “out-of-the-box” or “blue-sky” thinking and is an element of human problem-solving and ingenuity that today’s focused, single-minded and often obsessive AIs are unlikely to emulate in the near future.
This means AIs have to be taught to ensure that their solutions do not cause other problems, further down the line, in areas beyond those which they are designed to consider.
This includes learning not to step on the toes of other AIs. For example, in a smart city, it’s easy to imagine the effects of one AI system – managing security lighting, say – conflicting with another, such as regulating power usage.
These four key challenges which AI will have to overcome in the near future are certainly not insurmountable. But solutions will have to be implemented before AI will live up to its undoubtedly huge potential. In the case of most of them – generally those which will be solved by the advance of technology – that work is well underway. Others, though, will require human minds to come together and establish workable principles and codes of conduct, a process which could take a little more time.