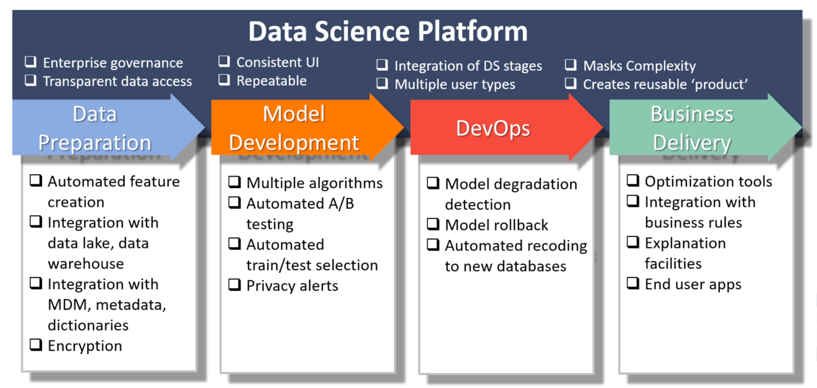
Introduction to Data Science Platforms
Data Science Platforms have revolutionized the way businesses operate by providing a comprehensive suite of tools for managing and analyzing large volumes of data. These platforms are designed to streamline the data processing and analytical workflow, making it easier for businesses to leverage insights in real-time. In this article, we explore the definition and architecture of data science platforms, their features, the importance of these platforms in business, and the different types of platforms available.
Definition of Data Science Platforms
Data Science Platforms are software tools that help organizations manage data processing and analytical workflows efficiently. They typically provide an integrated environment for data preparation, data analysis, and machine learning. These platforms automate the process of data collection, cleaning, and analysis, allowing businesses to derive insights from their data faster than traditional methods.
Overview of Data Science Platform Architecture
Data Science Platforms typically consist of three main components: data storage, data processing, and data analysis. These components work together to provide an integrated platform for businesses to manage their data workflow. Data storage provides a central repository for storing data, while data processing ensures that data is cleaned, normalized, and prepared for analysis. Finally, data analysis tools enable businesses to gain insights from their data using advanced analytical techniques such as machine learning and data visualization.
Features of Modern Data Science Platforms
Modern data science platforms come equipped with various features that make them indispensable to modern businesses. Some of these features include:
Data Integration and Preparation Capabilities
Data Science Platforms come equipped with tools for integrating data from various sources and cleaning and preparing data for analysis. This feature makes it easier for businesses to work with large, complex datasets, saving time and resources.
Machine Learning and AI Capabilities
Machine learning and AI capabilities are integral to modern data science platforms. These capabilities allow businesses to build predictive models that can be used to identify trends and patterns in data, helping businesses make informed decisions.
Data Visualization and Reporting Capabilities
Data Science Platforms come equipped with tools for data visualization and reporting, making it easier for businesses to present their findings to stakeholders. Visualization tools enable businesses to communicate complex data insights in a more accessible and understandable format.
Importance of Data Science Platforms in Business
Data Science Platforms have become increasingly important to businesses in recent years. Here are some of the main ways in which these platforms have helped businesses:
Improving Decision Making Processes
Data Science Platforms provide businesses with insights that can help them make informed decisions. These insights are derived from large datasets, and are used to identify patterns and trends that can impact business performance.
Optimizing Business Operations
Data Science Platforms can help businesses optimize their operations by providing insights into areas that could be improved. This can help businesses reduce costs and increase efficiency.
Increasing Revenue and Enhancing Customer Satisfaction
By leveraging insights provided by Data Science Platforms, businesses can improve their products and services, leading to increased revenue and enhanced customer satisfaction.
Different Types of Data Science Platforms
There are three main types of Data Science Platforms: Open Source Data Science Platforms, Enterprise Data Science Platforms, and Cloud-Based Data Science Platforms.
Open Source Data Science Platforms
Open Source Data Science Platforms are free, community-driven platforms that are great for small businesses or businesses that are just starting out. These platforms offer basic data preparation, analysis, and visualization tools.
Enterprise Data Science Platforms
Enterprise Data Science Platforms are designed for larger businesses and come equipped with more advanced analytics and machine learning capabilities. These platforms typically require a subscription or license fee.
Cloud-Based Data Science Platforms
Cloud-Based Data Science Platforms offer the flexibility of cloud infrastructure, making it easier for businesses to scale their data science capabilities. They are typically subscription-based and can be accessed from anywhere with an internet connection.
Key Considerations for Choosing a Data Science Platform
When choosing a data science platform, there are several key considerations to keep in mind that can ensure the success and efficiency of your data science projects. Here are some of the most important factors to consider:
Scalability and Performance
One of the most important considerations when choosing a data science platform is scalability and performance. As your data sets grow larger and more complex, you need a platform that can handle the increased volume and still deliver fast performance. Be sure to look for a platform that is designed to scale with your needs and can handle large, diverse, and varied data sets.
Data Security and Privacy
Data security and privacy are becoming more important than ever, especially with the increasing use of cloud-based data science platforms. Make sure the platform you choose has strong security features such as encryption, access controls, and data segregation. It should also be compliant with the latest security and privacy regulations.
Vendor Support and Community
Another important consideration is the vendor support and community. You want to choose a platform that has a strong support team that can provide timely and helpful assistance when you need it. You also want a platform with an active and engaged community that can help you solve problems and share best practices.
Integration of Data Science Platforms with Other Technologies
Integration is another important consideration when choosing a data science platform. A platform that integrates well with other technologies can streamline your workflows and improve the accuracy of your analyses. Here are some of the key considerations when it comes to integration:
Integration with Big Data Technologies
Big data technologies are an essential part of modern data science projects. Your platform should be able to integrate with popular big data technologies such as Hadoop, Spark, and NoSQL databases.
Integration with Business Intelligence Tools
Business intelligence tools can help you visualize and explore your data in new and powerful ways. Look for a platform that integrates well with popular BI tools such as Tableau, QlikView, and Power BI.
Integration with Cloud Platforms
Cloud platforms are becoming the go-to choice for data science projects due to their scalability and flexibility. Make sure your platform can take advantage of the latest cloud technologies such as AWS, Google Cloud, and Azure.
Future Trends in Data Science Platforms
Data science platforms are constantly evolving, with new trends and technologies emerging all the time. Here are some of the most important trends to keep an eye on in the coming years:
Increased Automation and Democratization
Automation and democratization are two key trends that will shape the future of data science platforms. As automation technologies such as machine learning and AI become more prevalent, data science platforms will become more accessible to non-technical users.
Growing Importance of Explainable AI
Explainable AI is becoming more important as the use of AI becomes more widespread. Data science platforms that can provide insights into the decision-making processes of AI models will become increasingly valuable.
Convergence of Data Science and DevOps
Finally, data science and DevOps are converging, with data science platforms incorporating more DevOps-like features such as continuous integration and continuous deployment. This trend will accelerate as more organizations adopt data-driven decision-making.In conclusion, data science platforms offer a powerful solution for organizations looking to extract meaningful insights from their data to drive business outcomes. By providing a unified environment for data science workflows, these platforms can help streamline processes, enhance collaboration, and improve decision-making. When choosing a data science platform, it’s important to consider factors such as scalability, security, and vendor support. As technology continues to evolve, we can expect data science platforms to become even more automated and democratized, enabling organizations of all sizes to leverage the power of data science.
FAQ
What is a data science platform?
A data science platform is a software solution that enables organizations to manage the entire data science workflow, from data preparation to model deployment. These platforms provide a unified environment for data scientists and other stakeholders to collaborate and extract insights from data.
What are the benefits of using a data science platform?
Using a data science platform can help organizations streamline their data science workflows, enhance collaboration between teams, and improve decision-making processes. These platforms also provide a range of features and capabilities, such as data integration, machine learning, and data visualization, that can help organizations extract meaningful insights from their data.
What are some key factors to consider when choosing a data science platform?
When choosing a data science platform, it’s important to consider factors such as scalability, security, and vendor support. Other important considerations include the types of data sources and formats supported by the platform, the platform’s user interface and ease of use, and the availability of pre-built models and algorithms.
What are some future trends in data science platforms?
As technology continues to evolve, we can expect data science platforms to become more automated and democratized, enabling organizations of all sizes to leverage the power of data science. We can also expect to see a growing emphasis on explainable AI, as well as increased convergence between data science and DevOps.