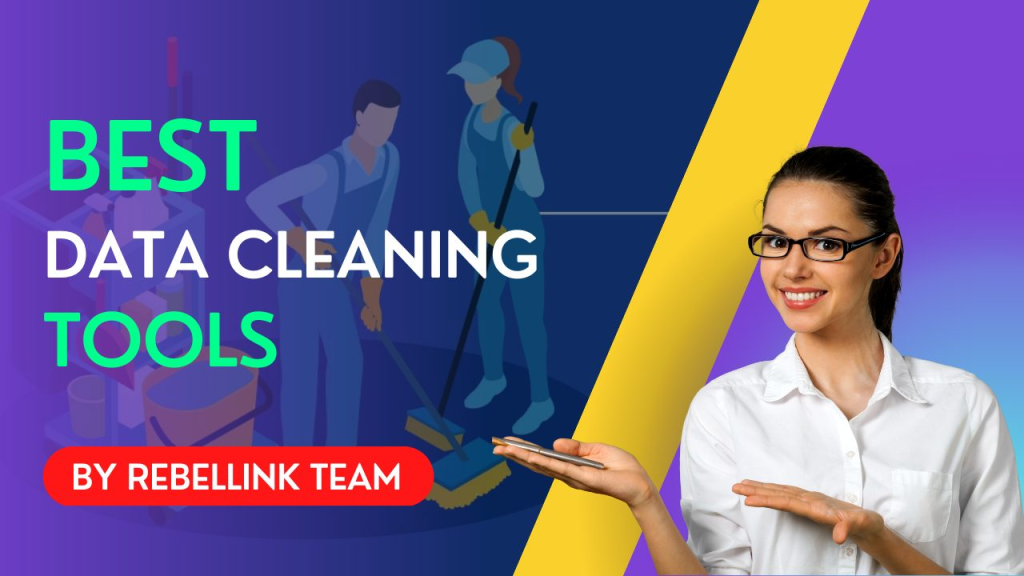
Introduction to Data Cleaning Tools
In today’s data-driven world, businesses and organizations generate vast amounts of data every day. This data can come in various forms and from multiple sources, making it challenging to manage and analyze. Data cleaning is the process of detecting and correcting errors, inconsistencies, and inaccuracies in data. It is a critical step in data preparation that ensures the data is of high quality, reliable, and consistent. In this article, we will explore how data cleaning tools can help organizations automate this process and ensure data accuracy.
What is Data Cleaning?
Data cleaning involves identifying and correcting errors and inconsistencies in data to ensure it is accurate, reliable and consistent. The process involves several steps such as removing duplicates, correcting typos, standardizing fields, and dealing with missing or inconsistent data. Data cleaning can be a time-consuming process, especially when dealing with large datasets. However, it is essential to ensure that any insights or decisions made from the data are based on accurate information.
Why is Data Cleaning Important?
Data cleaning is essential for several reasons. Firstly, it ensures data accuracy and consistency, which is crucial for making informed decisions. Secondly, it saves time and resources by eliminating the need to manually clean large datasets. Finally, it helps organizations comply with regulations and industry standards that require accurate and consistent data.
Types of Data Cleaning Tools
Data cleaning tools come in different forms, including open source, proprietary, and cloud-based solutions.
Open Source Data Cleaning Tools
Open source data cleaning tools are software that is freely available and can be modified, distributed and used without any restrictions. Open source tools are a popular choice since they are free and offer a high degree of customization. Examples of open source data cleaning tools include OpenRefine and Datapackage.
Proprietary Data Cleaning Tools
Proprietary data cleaning tools are software developed by commercial vendors and sold for a fee. Proprietary tools often offer more features and support than open-source alternatives and are a popular choice for enterprise-level organizations. Examples of proprietary data cleaning tools include Informatica and Talend.
Cloud-Based Data Cleaning Tools
Cloud-based data cleaning tools are software that is hosted and accessed over the internet. These tools offer the advantage of being accessible from anywhere, and there is no need to install or maintain any software. Examples of cloud-based data cleaning tools include Trifacta and Google Refine.
Advantages of Using Data Cleaning Tools
Using data cleaning tools offers several advantages, including:
Improved Data Accuracy
Data cleaning tools help detect and correct errors, inconsistencies, and inaccuracies, ensuring that the data is of high quality and accurate.
Reduced Errors and Duplicates
Data cleaning tools eliminate manual data entry errors and duplicate records, reducing the risk of inaccurate data.
Increased Efficiency and Productivity
Data cleaning tools automate the time-consuming process of cleaning and standardizing data, freeing up valuable time and resources.
Top Data Cleaning Tools in the Market
Several data cleaning tools are available in the market, each with unique features and capabilities. Here are some of the top data cleaning tools in the market:
Trifacta
Trifacta is a cloud-based data cleaning tool that offers a range of features, including data profiling, data wrangling, and data quality assurance. It is highly customizable and can handle complex data formats.
OpenRefine
OpenRefine is an open-source tool that offers powerful data cleaning and transformation capabilities. It can handle large datasets and offers extensive support for data exploration and filtering.
Talend Data Preparation
Talend Data Preparation is a proprietary tool that offers data profiling, cleaning, and enrichment capabilities. It can handle various data sources, including structured and unstructured data, making it ideal for enterprise-level organizations.
Data Ladder
Data Ladder is a proprietary data cleaning tool that offers a range of features, including deduplication, data profiling, and standardization. It is easy to use and offers real-time data cleaning and validation.
Criteria for Selecting the Best Data Cleaning Tool
Data cleaning tools are essential for ensuring data accuracy and optimizing data-driven decision-making processes. Choosing the right data cleaning tool is crucial for successful data cleaning. Here are some essential criteria to consider before selecting the best data cleaning tool:
Usability and User-Friendliness
A user-friendly data cleaning tool is essential because most of the data cleaning tasks are time-consuming and require continuous attention. A tool that has a simple and intuitive interface is preferred because it will save time and reduce errors.
Data Compatibility and Integration
The compatibility of a data cleaning tool with different data sources and ability to integrate with various data analysis tools is a critical factor. A tool that can import and export data from different file formats is preferred because it offers more flexibility.
Functionality and Features
The functional capabilities of a data cleaning tool is a significant consideration. Data cleaning tools should have a wide range of features that include data profiling, data parsing, and data standardization. The tool should also have advanced functionalities such as fuzzy matching, duplicate detection, and advanced data visualization.
Best Practices for Using Data Cleaning Tools
Using data cleaning tools comes with some best practices that help to increase efficiency and effectiveness. Here are some best practices for using data cleaning tools:
Establish Data Quality Goals
Establishing data quality goals before beginning the data cleaning process is essential. Setting the quality goals will help to prioritize data cleaning tasks that have the most significant impact on data quality.
Use Standardized Naming Conventions
Using standardized naming conventions ensures consistency and accuracy in data processing. Naming conventions should be easy to understand by all parties involved in the data cleaning process.
Document Data Cleaning Processes
Documentation is crucial in data processing. Documenting data cleaning processes helps to keep track of the data cleaning steps taken, enabling easy identification of what has been done so far and areas that require more attention.
Challenges Encountered while Cleaning Data Using Tools
Data cleaning comes with its own set of challenges. Here are some of the challenges that are faced while cleaning data using tools:
Handling Large Data Volumes
Handling large volumes of data during the cleaning process can be challenging. The data cleaning tool should have the capability to handle large data volumes.
Quality of Data
The quality of data varies from source to source, and it can be difficult to maintain data consistency, completeness, and accuracy.
Handling Unstructured Data
Unstructured data is challenging to handle because it lacks a predefined structure. Data cleaning tools should have advanced functionalities that can handle unstructured data.
Future of Data Cleaning Tools
The future of data cleaning tools looks promising, with more technological advancements expected. Here are some of the future trends expected in data cleaning tools:
Artificial Intelligence and Machine Learning
Artificial intelligence and machine learning are expected to play a crucial role in data cleaning tools. AI and ML algorithms can automate data cleaning processes and reduce errors.
Increased Integration with Big Data Technologies
Data cleaning tools will be more integrated with big data technologies like Hadoop, Spark, and NoSQL databases. The integration will enable efficient processing of large data volumes.
Enhanced Automation and Reduced Human Intervention
Data cleaning tools will be more automated, reducing the need for human intervention. Automated tools will save time, reduce errors, and increase efficiency.In conclusion, data cleaning tools are essential resources for organizations looking to improve the quality and accuracy of their data. By adopting data cleaning tools, organizations can reduce errors and inconsistencies, improve data accuracy, and increase efficiency and productivity. However, selecting the best data cleaning tool and using it effectively requires careful consideration of different factors, including functionality, usability, and compatibility with existing data systems. As technology continues to advance, we can expect to see more sophisticated data cleaning tools that leverage artificial intelligence and machine learning to provide even more efficient and effective data cleaning solutions.
FAQs
What is data cleaning?
Data cleaning is the process of identifying and correcting errors, inconsistencies, and inaccuracies in large datasets. It involves a range of activities, from removing duplicate records to standardizing data formats and ensuring consistency in data entry.
What are some common challenges encountered while cleaning data using tools?
Some of the common challenges encountered while cleaning data using tools include handling large data volumes, ensuring the quality of data, and handling unstructured data. Other challenges include dealing with incomplete or missing data, dealing with data in different formats, and ensuring compatibility with existing data systems.
What are some best practices for using data cleaning tools?
Some best practices for using data cleaning tools include establishing clear data quality goals, using standardized naming conventions, documenting data cleaning processes, and ensuring the security and confidentiality of data. It is also important to regularly review and update data cleaning processes to ensure that they remain effective.
Why should organizations invest in data cleaning tools?
Organizations should invest in data cleaning tools because they help to improve the accuracy and reliability of data, reduce errors and inconsistencies, and increase efficiency and productivity. By using data cleaning tools, organizations can ensure that their data is of high quality and can be used to make informed decisions that drive business success.