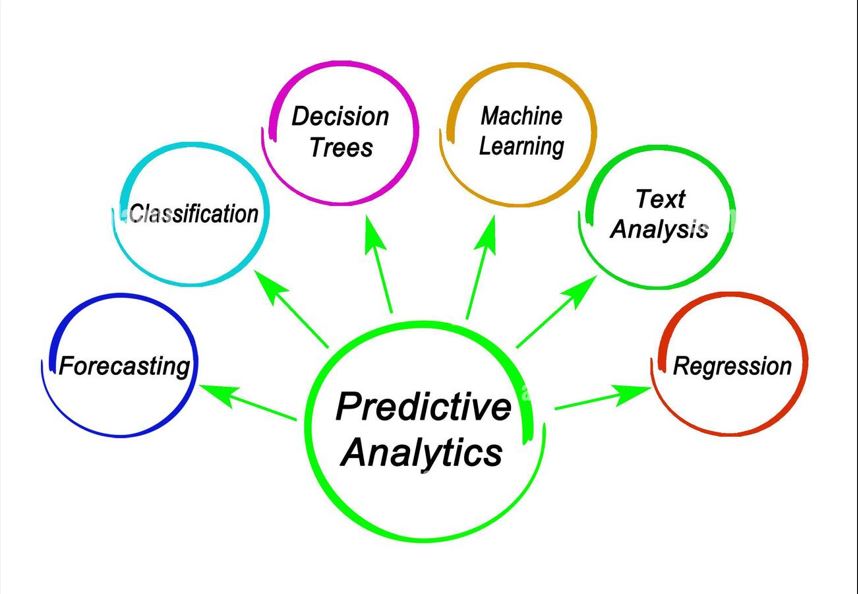
Introduction to Predictive Analytics Tools
As businesses continue to collect vast amounts of data, it becomes increasingly challenging to make informed decisions that drive growth and improve customer experience. Predictive Analytics Tools can help bridge this gap by analyzing data patterns, identifying trends, and forecasting outcomes. In this article, we’ll explore what predictive analytics is, the different types of predictive analytics tools, their benefits, as well as some of the challenges and limitations that organizations might face when using them.
What is Predictive Analytics?
Predictive Analytics is a branch of advanced analytics that utilizes statistical techniques, machine learning algorithms, and data mining to predict future events or outcomes. It involves analyzing historical data to uncover patterns and relationships, which are then used to make predictions about future events. These predictions can be used to optimize business processes, identify potential risks or opportunities, and improve decision-making.
Overview of Predictive Analytics Tools
Predictive Analytics Tools can be broadly categorized into three types: Machine Learning-Based, Rule-Based, and Model-Based. Machine Learning-Based tools are driven by artificial intelligence and develop insights and models based on data inputs. Rule-Based tools use business rules and specific criteria to generate insights, and Model-Based tools create models based on historical data to predict future outcomes.
Types of Predictive Analytics Tools
Machine Learning-Based Predictive Analytics
Machine Learning-Based Predictive Analytics leverages Artificial Intelligence and Machine Learning algorithms to develop insights, models and forecasts based on large datasets. It can analyze data patterns, identify trends and predict outcomes in real-time, making it a powerful tool for data-driven decision-making.
Rule-Based Predictive Analytics
Rule-Based Predictive Analytics uses a set of business rules and predefined criteria to analyze data and generate insights. This type of predictive analytics tool is particularly useful when making decisions based on pre-established criteria or when particular outcomes need to be identified.
Model-Based Predictive Analytics
Model-Based Predictive Analytics uses existing data to create models that can be used to make predictions about future events or outcomes. These tools rely on data mining and statistical modeling and are typically more accurate than Rule-Based predictive analytics tools.
Benefits of Using Predictive Analytics Tools
Improved Decision Making
Predictive Analytics tools can help organizations make more informed decisions by providing insights based on historical data and real-time analysis. These tools can help identify hidden patterns and relationships that might not be visible through traditional analytics methods, enabling organizations to make data-driven decisions.
Increased Efficiency and Cost Savings
Predictive Analytics tools can help organizations optimize business processes by identifying areas of inefficiency and providing recommendations for improvement. This can lead to significant cost savings and increased operational efficiency.
Enhanced Customer Experience
Predictive Analytics tools can help organizations gain valuable insights into customer behavior and preferences. This can be used to create targeted marketing campaigns and personalized product recommendations, which can ultimately improve customer satisfaction and retention.
Challenges and Limitations of Predictive Analytics Tools
Data Quality and Quantity
Predictive Analytics tools rely on historical data to make predictions about future outcomes. Therefore, the accuracy of these predictions can be limited by the quality and quantity of data available. Data pre-processing efforts are necessary to ensure that the data is accurate and of high quality.
Expertise and Resource Constraints
Effective use of predictive analytics tools require skilled personnel and resources. Organizations must invest in training employees on how to use these tools and hire experts to develop and implement predictive models.
Privacy and Ethical Concerns
As predictive analytics tools rely on personal data, there are concerns about privacy and ethical implications. Organizations must be transparent and ensure they comply with data protection laws and regulations, particularly when handling sensitive data.
How to Choose the Right Predictive Analytics Tool for Your Business
When it comes to choosing the right predictive analytics tool for your business, there are several factors to consider. One of the most important is identifying your business needs and goals. What specific problems are you looking to solve through predictive analytics? What outcomes are you hoping to achieve?
Another important factor is evaluating the features and capabilities of different predictive analytics tools. These can vary widely depending on the tool, so it’s important to do your research and understand what each tool can offer your business.
Finally, you’ll need to consider how the tool will integrate with your existing systems. This can be a complex process, but it’s essential to ensure that your new predictive analytics tool can seamlessly integrate with your current infrastructure.
Best Practices for Implementing Predictive Analytics Tools
Once you’ve chosen a predictive analytics tool, it’s time to implement it effectively. There are several best practices to keep in mind during this process.
First, it’s important to define clear objectives and metrics to ensure that you’re using the tool to its full potential. This can help you measure success and make adjustments as needed.
Data quality and availability are also crucial to the success of your predictive analytics implementation. Ensure that your data is accurate, relevant, and readily available to avoid any delays or errors.
Finally, establishing governance and oversight is essential to ensuring that your predictive analytics implementation is running smoothly. This can include setting up a team to manage the tool, creating rules and guidelines for its use, and regularly monitoring its performance.
Case Studies: Real-Life Examples of Successful Predictive Analytics Implementations
Real-life examples of successful predictive analytics implementations can provide valuable insights into how to use these tools effectively. Here are three examples from different industries:
Healthcare Industry
One healthcare organization used predictive analytics to identify patients who were at high risk of being readmitted to the hospital. By analyzing patient data, the organization was able to proactively reach out to these patients and provide additional care and support, resulting in a significant reduction in readmissions and cost savings.
Retail Industry
A retail company used predictive analytics to optimize its pricing strategy. By analyzing historical sales data, the company was able to identify products that were likely to sell well at different price points and adjust its pricing accordingly. This resulted in increased sales and profitability.
Financial Services Industry
A financial services company used predictive analytics to identify high-risk loans before they became delinquent. By analyzing historical loan data, the company was able to develop a model that accurately predicted which loans were most likely to default. This allowed the company to take proactive measures to prevent delinquencies and reduce losses. In conclusion, predictive analytics tools have revolutionized the way businesses make decisions. By leveraging data to predict future outcomes, organizations can gain a competitive advantage and stay ahead of the curve. While there are challenges and limitations to the use of predictive analytics tools, with careful planning and implementation, businesses can optimize their use and reap the benefits. By following best practices and learning from real-life case studies, organizations can harness the power of predictive analytics tools to drive better business outcomes and achieve success.
FAQ
What kind of businesses can benefit from using predictive analytics tools?
Predictive analytics tools can benefit businesses of all sizes and industries. Any organization that relies on data to make informed decisions can benefit from using predictive analytics tools. This includes healthcare, retail, finance, and many other industries.
What are some examples of predictive analytics tools?
Some popular predictive analytics tools include SAS Predictive Analytics, IBM SPSS Modeler, Rapid Miner, and Microsoft Azure Machine Learning. There are many other tools available, and the best one for your business will depend on your specific needs and goals.
What are the benefits of using predictive analytics tools?
The benefits of using predictive analytics tools include improved decision making, increased efficiency and cost savings, and enhanced customer experience. By analyzing data to predict future outcomes, organizations can act with greater confidence and agility, and make decisions that drive business success.
What are the challenges of using predictive analytics tools?
Some of the challenges of using predictive analytics tools include data quality and quantity, expertise and resource constraints, and privacy and ethical concerns. However, with careful planning and implementation, these challenges can be overcome, and businesses can effectively leverage predictive analytics tools to drive better business outcomes.